Will students soon be graded using a neural test?
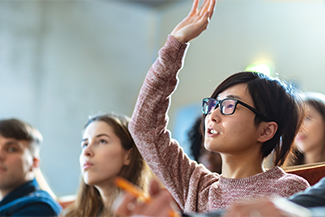
Researchers at Dartmouth College and Harvard University started from a simple assumption: when an individual acquires new knowledge, it has to be represented somewhere in the brain. If this is the case, these representations should be illustrated through new patterns of brain activity. The scientists then developed a protocol to try to measure a person’s degree of knowledge on a subject by looking at his or her neural data and assigning what they call a “neural score.” The goal of the research was to develop an automatic learning algorithm to predict a student’s acquired conceptual knowledge.
The scientists recruited 28 participants (M = 20.71 years; 16 women) for the experiment. Half of the subjects had no engineering or physics experience (the “novice group”); the others (the “student group”) were about to finish (or had completed) a degree in mechanical engineering.
At the beginning of the experimental protocol, the participants were given two standard (multiple choice) tests to assess their knowledge of physical and mechanical engineering. The “students” were much better than the “novices,” correctly answering 50.2% (vs 16.9%) and 79.3% (vs 35.9%) of the questions. Next, the participants were placed in an fMRI scanner and were shown 24 photographs of real-world structures (bridge, lamppost, various buildings, etc.) and were asked to analyze how the structures were built. The aim was to evaluate their understanding of Newtonian forces (how the forces of a given structure are balanced so it stays standing). Next, the participants had to tell whether a diagram with arrows showing the forces at work on a structure was correct or not. At this stage, the “students” succeeded 75% of the time, while the “novices” answered correctly 53.6%. of the time.
Following the fMRI sessions and using a new multivariate neuroimaging approach (based on informational network analysis), the researchers were able to obtain a “neural score” from the activity patterns in the brain. This score could then be used to predict individual differences in the participants’ performance on the various tasks. Among the scores calculated, the researchers were particularly interested in the “informational network” score. To validate it, the neural score of each student was compared with his or her performance on the three conceptual knowledge tests. The results showed that the higher the neural score, the better the student did on the tests.
In order to identify cortical areas, the researchers also represented this neural score by projecting the results of all the participants onto a kind of surface map of the brain. The engineering students' conceptual knowledge was associated with patterns of activity in several brain regions, including the dorsal frontoparietal network (which helps enable spatial cognition), and regions of the ventral occipitotemporal cortex (which is involved in visual object recognition).
In conclusion, this study is one of the first to formalize how knowledge that’s being acquired is represented in the brain. While subject to a number of reservations, an algorithm that’s capable of assigning a “neural score” might be useful in assessing whether a learner understands the course material. This would be helpful in adjusting teaching methods. But for now, we’re still in the experimental stage; the authors are curious to see whether the accuracy of their “neural score” can be generalized to subjects outside of math and physics…
Source: Joshua S. Cetron, Andrew C. Connolly, Solomon G. Diamond, Vicki V. May, James V. Haxby & David J. M. Kraemer, “Decoding individual differences in STEM learning from functional MRI data”, in Nature Communications, vol. 10, May 2019